The 2030 Vision for Underwater Autonomy
Jorge L. Hurtado, PhD Research Analyst and Assistant Editor
Autonomous underwater platforms, in tandem with Artificial Intelligence, have soared to new heights in recent years. These platforms operate independently, navigating the depths and executing missions without the need for constant human oversight. The top-tier of these platforms showcase a range of remarkable capabilities, from collecting diverse information types vital for scientific, commercial, and military endeavors.
Nevertheless, achieving full autonomy presents a substantial hurdle according to a recent PreScouter report. To see major improvements toward the complete autonomy of underwater platforms, a holistic approach is crucial in addressing these technical limitations and capabilities. It requires substantial investments, notably in updating training data for underwater settings, refining labeled image data, and acquiring essential information like SONAR, all demanding considerable resources.
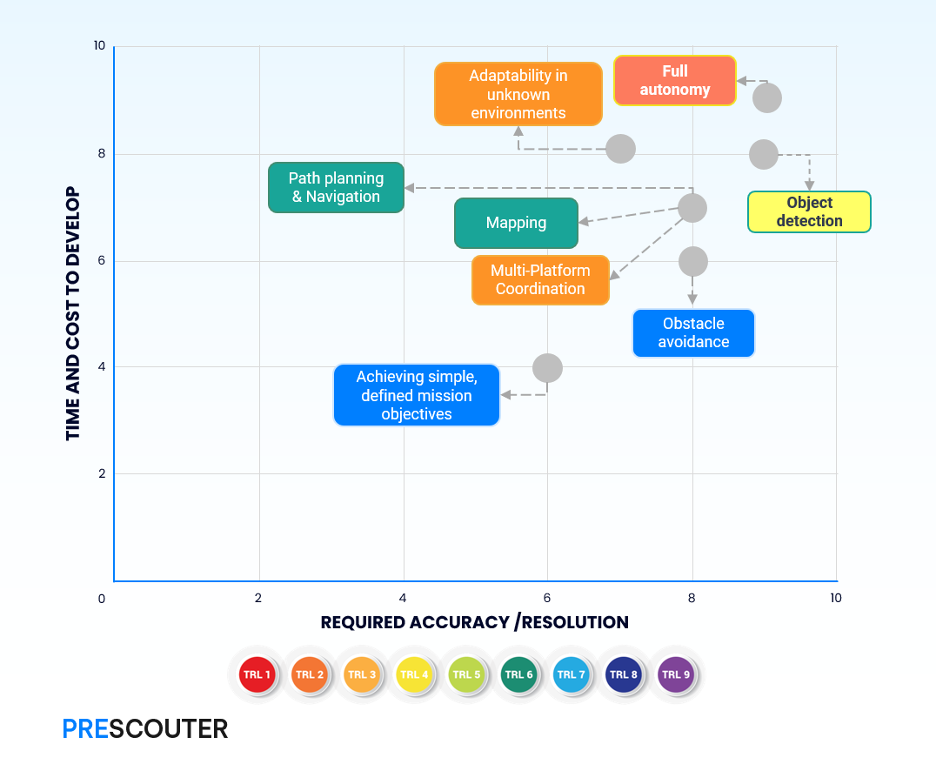
As seen in the figure above, the TRLs show that various technological aspects of underwater platform development have progressed from conceptual stages (TRL 3) through component validations (TRL 4) and the creation of functional prototypes (TRL 6 and 7). TRL 7, denoting operational testing, marks significant progress towards practical application. However, the SME analysis, considering the time and costs required to achieve full autonomy in terms of accuracy and resolution, suggests that we still have a considerable distance to go.
The progress of autonomous underwater platforms faces long-term developmental limitations
In the short-term, underwater autonomous platforms are expected to grasp the intricate connections between objects and events in both spatial and temporal dimensions. In addition, autonomous systems should be equipped with machine learning prowess to make informed decisions by selecting actions that optimize outcomes.
To meet these expectations, we need to solve the problem that sensors underwater face limitations in range, resolution, and visibility. Battling with high noise levels and uncertainties, they make it tough for AI systems operating underwater to crunch and decipher the data they gather.
The demands on AI systems are immense, requiring hundreds of terabytes of data to accomplish tasks effectively. However, the journey to acquire and label such data is a costly and time-consuming endeavor.
Picture this: image labeling alone averages $2.50 per image, and we're talking potentially hundreds of thousands of images required to truly enhance these capabilities. Moreover, the challenges in obtaining and utilizing SONAR data tailored for underwater settings are profound. The existing methods struggle to overcome persistent limitations like overdrifting, noise interference, and the haunting presence of shadows.
To even begin to move the needle towards achieving autonomy in underwater environments, we're talking about a minimum investment ranging from $5 to $10 million. That's just to update the training data essential for underwater applications, particularly the labeled image data.
Mind you, this doesn't even cover the expenses of gathering this data or leveraging more relevant sources like SONAR in the initial phase. According to experts interviewed by PreScouter, we're looking at a ballpark figure of $100 million to push the current state-of-the-art platforms to achieve full autonomy. It's a monumental challenge, but with strategic investments and groundbreaking innovations, we pave the way for a future where autonomous underwater platforms redefine what's possible.
Two key gaps are addressing "noisy" data and boosting latencies by 30X
Aquatic environments present unique hurdles for AI systems due to its interference with electromagnetic wave propagation, causing disruptions in signals. To navigate these challenges effectively, there's an imperative to refine communication protocols, amplify bandwidth, curtail latency to less than 10 milliseconds (a considerable reduction from the 300-400 milliseconds observed in autonomous cars), and fortify signal resilience. These measures are crucial for establishing seamless and robust communication among diverse entities.
High-level autonomous systems need to manage data with significant noise and uncertainty. Consequently, there exists an urgent demand to ameliorate system latencies by a staggering 30-fold. This enhancement is pivotal in surmounting the complexities posed by fluctuating data quality and uncertainties, paving the way for more efficient and adaptive underwater systems.
Continuous advancements in hardware will boost the processing capabilities of underwater AI engines.
Better-performing autonomous architectures and edge computing are crucial. The ongoing progress in hardware is set to supercharge the processing power of AI engines navigating underwater landscapes. To reach the pinnacle of 1000 TOPS for achieving complete autonomy, it requires a unified architecture housing 25 cutting-edge edge computing chips.
Resolving concerns surrounding computational hardware is pressing for lightning-fast real-time data processing. This demands an upgrade in sensor technology and the adoption of powerful tools like FPGAs. The fusion of deep learning with SONAR, coupled with the integration of edge computing, holds the key to overcoming latency challenges and maximizing the potential of these platforms.
Moreover, to attain sustained autonomy, models require a minimum of a 7 TRLs advancement. Despite progress made with the use of YOLO v5 and Mask R-CNN, along with methods such as NASA's Jet Propulsion Lab's Model-Driven Engineering and domain-specific languages (DSLs), reaching real-world complete autonomy demands a significant 7 TRLs leap, according to Valencian Research Institute for Artificial Intelligence’s analysis.
Though the journey towards achieving autonomy in underwater platforms is still in its early stages, notable strides are being made by prominent leaders on a global scale. To meet the burgeoning demands of commercial and defense sectors worldwide, and to attain complete autonomy, further research and development efforts are imperative. Notably, major players like NVIDIA have set their sights on addressing this pressing need.
A 10x increase in patent applications for underwater autonomy/AI in the last 7 years
Over the past seven years, there has been a remarkable surge, almost a tenfold increase, in patent applications related to underwater autonomy and AI.
This surge underscores the burgeoning interest and innovation in underwater autonomy. PreScouter analysis of intellectual property highlights the broadening scope of applications encompassing surveillance, unmanned vehicles, and cutting-edge sensors. The advancements in specialized chips and neural networks serve as linchpins in the quest for achieving autonomy in this domain.
The journey forward to full autonomy of underwater platforms
In the mid-term trajectory, our focus lies on transforming underwater mapping into a cutting-edge domain. We're dedicated to employing an array of diverse sensor methods and pioneering strategies, envisioning a future where our systems boast real-time spatial awareness updates. These updates are pivotal, providing AI engines with the necessary information for precise navigation in challenging underwater environments.
Fundamentally, the success of autonomous underwater platforms hinges on possessing precise and extensive underwater mapping, a task attributed to SONAR technology. To generate meaningful information, SONAR requires the collection of substantial mapping data on an hourly basis, ranging from several gigabytes to tens of gigabytes. With this pre-processed information AI engines will have a strong support in guiding autonomous navigation and facilitating exploration. Underwater mapping serves as a critical resource, furnishing highly accurate topological, semantic-based, and geometrical maps of any environment, enabling the identification of resources even under any extreme settings.
So far the latest computer vision algorithms are gearing up to revolutionize predictive capabilities. Real-time predictions can forecast forthcoming actions and locations by analyzing and learning from past behaviors, propelling underwater platforms into an era of proactive decision-making.
In the longer term, the critical hurdles will demand groundbreaking solutions. Navigating through underwater complexities is a key challenge - conceptually AI systems need to swiftly adapt and make real-time decisions. From maneuvering through intricate obstacles to navigating through ever-changing environmental conditions like currents, temperature variations, pressure fluctuations, and limited visibility, AI engines must react instantly and accurately to ensure safe and efficient underwater navigation.
Keeping AI systems reliable for extended periods in remote underwater locations poses intricate engineering challenges. It's about ensuring these systems remain robust and efficient over time in challenging conditions, demanding meticulous attention to reliability and continuous upkeep - a challenge expected to overcome in the long run.
Another long-term challenge is to be able to design AI engines that not only adapt but also learn in uncharted or rapidly transforming environments. This shall be a significant ambition. We aim to equip these systems with the ability to thrive and excel amidst novel or swiftly changing conditions, paving the way for a new level of adaptability and learning capabilities.
It is expected that in the long run, AI systems should evolve and adapt dynamically, learning and refining their models in real time. Imagine an AI system capable of forming hypotheses about the aquatic environment around it, enhancing its understanding and adaptability with each passing moment.
Furthermore, we're gearing up for a paradigm where our systems will exhibit extended-term reasoning capabilities spanning years. To achieve this, we're cultivating more abstract reasoning capabilities, enabling our systems to navigate and comprehend complex scenarios over prolonged periods, marking a significant leap forward in their cognitive abilities.
Summing up, underwater autonomous platforms integrated with AI engines hold immense potential across various sectors. Yet, overcoming current limitations remains pivotal to achieving full autonomy.
-
Lack of ample data remains a significant challenge, particularly in training AI engines for complex tasks such as object detection. In the demanding underwater environments, data collection grapples with hurdles due to the harsh conditions, ushering in noise and interference.
-
The complexities are multifaceted. Implementing AI engines, especially with restricted computing and cloud access, presents intricate challenges. Among the most pressing unresolved tasks remain sensor data handling and processing, posing as formidable hurdles in our pursuit of progress.
-
The cost factor is undeniable. Developing and deploying AI engines incurs significant expenses encompassing R&D, specialized hardware, sensor components, communication equipment, power sources, and more. Again, it’s a costly affair demanding substantial investment.
-
Time is a valuable asset in this domain. Training AI engines for intricate tasks demands extensive data, prolonging the process. In the underwater realm, data collection faces persistent challenges owing to the unforgiving conditions.
-
Diving deeper into data complexities, training AI with labeled data proves to be an expensive endeavor. Efforts are underway to address this hurdle through unsupervised data processing, yet the road ahead remains intricate.
-
Navigating environmental heterogeneity presents its own set of challenges. Autonomous Underwater Vehicles (AUVs) traverse diverse environments, making it daunting to train AI engines to perform seamlessly across all conditions. Underwater environments across different regions, be it Europe, the Americas, or Asia, exhibit distinctive characteristics, hence demanding region-specific data for optimum performance. The variability in environments, spanning freshwater to marine realms and different depths, adds layers of complexity.
-
Furthermore, the variety of sensors employed by AUVs, ranging from cameras to sonar and radar, complicates AI training for effective multi-sensor utilization. Coordinating and interpreting data across these diverse sensors presents a logical puzzle for AI engines.
-
Engaging various stakeholders is crucial, yet it also introduces complexities. Achieving AI consensus among the diverse stakeholders involved in AUV autonomy remains a challenge. Limited collaboration among research institutions, industry partners, and experts in engineering and design further amplifies the complexity.
While achieving full autonomy in underwater platforms may seem like a distant goal at this stage, it's important to note that these challenges are not insurmountable. Evidence for such advances are out there, human-based science is based on dedicated collaboration and the pursuit of innovative solutions. Thus, we will undoubtedly overcome these hurdles and provide autonomous underwater platforms with the main key aspect: full autonomy.
About the Author:
Jorge L. Hurtado, PhD Research Analyst and Assistant Editor
Jorge, a Research Analyst and Editor Assistant at PreScouter, helps provide clients with high-quality information and analysis on disruptive technologies, aiding companies find new markets and stay competitive.